Enabling Accurate and High-Throughput Kinetics Predictions via Message Passing Neural Networks
Author(s)
Spiekermann, Kevin A.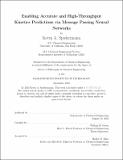
DownloadThesis PDF (45.35Mb)
Advisor
Green, William H.
Terms of use
Metadata
Show full item recordAbstract
Quantitative estimates for kinetic properties, namely reaction barrier heights and reaction energies, are essential for developing kinetic mechanisms, predicting reaction outcomes, and optimizing chemical processes. While ab initio methods, such as quantum chemistry, can be incredibly useful for providing accurate kinetic data, their high computational cost severely limits their utility for large-scale applications. High-quality experimental data is often even more rare, and such approaches are less amenable to exploring the vastness of chemical space due to monetary cost, time, and safety considerations. Modern machine learning (ML) techniques offer a promising option since they can quickly provide estimates to narrow the search space for more expensive ab initio or experimental methods. Unfortunately, the paucity of reliable quantitative chemical reaction data to train such models has presented a major hindrance for these data-driven approaches.
Here, this thesis focuses on the intersection of ML and quantum chemistry with the goal of enabling automatic high-fidelity predictions of kinetic parameters. The novel contributions can be grouped into three main categories:
1. Large-scale dataset generation, with an emphasis on high-quality methods and reaction diversity. Although much of the presented work studies reactions in the gas phase, this thesis also contributes a large dataset calculated in many popular solvents.
2. Train various ML models to quickly predict accurate kinetic parameters, which avoids the challenging task of finding transition state structures. Importantly, these models operate on simple input representations and hence are ideal for automated, high-throughput applications.
3. Provide best-practice guidelines and an open-source software package to improve the status quo of ML for chemistry research.
The contributions from this thesis, and from similar work, will be essential for modern high-throughput workflows and the future of automated predictive chemistry.
Date issued
2023-09Department
Massachusetts Institute of Technology. Department of Chemical EngineeringPublisher
Massachusetts Institute of Technology