The Effects of Pre-Training and Fine-Tuning CLIP with Domain-Specific Data
Author(s)
Wang, Jialan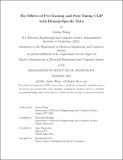
DownloadThesis PDF (13.36Mb)
Advisor
Matusik, Wojciech
Daptardar, Ajay
Terms of use
Metadata
Show full item recordAbstract
Mercari is an online two-sided marketplace that allows users to both sell and purchase items. To create the most efficient item listing process for the sellers and bring the most relevant items to the buyers, Mercari utilizes a pre-trained model called Contrastive Language-Image Pre-training (CLIP), famed for its exceptional zero-shot performances, to support the auto-filling feature for item listing and similar items recommendation. As this model is pre-trained on a general dataset gathered from the Internet, which likely does not have the same data distribution as Mercari’s data and results in non-optimal performance, we would like to explore the possibility of pre-training or fine-tuning CLIP with Mercari’s data to improve its performance within Mercari’s data domain. We explore various training strategies to understand the effects of each and determine the most effective strategy. Our best-performing and most space-efficient model achieves a brand prediction top-1 accuracy of 89.34% with 49.89% coverage and a category prediction accuracy of 78.02% with 69.62% coverage, significantly outperforming the current zero-shot CLIP in brand prediction and marginally in category prediction. Moreover, it achieves this with an embedding size that is half of that of the original CLIP.
Date issued
2023-09Department
Massachusetts Institute of Technology. Department of Electrical Engineering and Computer SciencePublisher
Massachusetts Institute of Technology