Machine Learning Approaches for Characterizing ALS Disease Progression
Author(s)
Ramamoorthy, Divya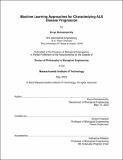
DownloadThesis PDF (16.19Mb)
Advisor
Fraenkel, Ernest
Terms of use
Metadata
Show full item recordAbstract
Amyotrophic Lateral Sclerosis (ALS) is a fatal neurodegenerative disease that is complex in its onset, pattern of spread, and disease progression. This heterogeneity makes it challenging to identify potential therapeutics and to evaluate their effectiveness in slowing progression. At the same time, a better understanding of the heterogeneity of ALS might help identify environmental or genetic modifiers of disease that could be targeted therapeutically. Despite the importance of accurately modeling ALS progression, current computational methods fail to capture the complexity of disease progression.
In this thesis, I describe machine learning approaches to characterizing disease progression in ALS. I first present the development of a Mixture of Gaussian Processes model to learn clusters of ALS disease progression from sparse longitudinal clinical data. I show that our learned trajectories are robust to sparse data, and correlate with alternate clinical measures such as survival. I also demonstrate applications of the method to other neurodegenerative diseases, including Alzheimer’s Disease and Parkinson’s Disease.
Next, I interrogate molecular features that correlate with clinical progression patterns. I longitudinally profile untargeted metabolomics and phosphorylated neurofilament heavy chain for a cohort of 283 individuals across 687 visits. Our results show that a PLSR model can be used to estimate disease severity, including ALSFRS-R and Vital Capacity, from metabolite concentrations. I also show that the distributions of neurofilament levels vary between ALS progression patterns.
Together, these results advance our understanding of disease progression in ALS, with critical implications for clinical trial analysis. These results also advance our biological understanding of the complex molecular changes that are associated with the disease.
Date issued
2022-05Department
Massachusetts Institute of Technology. Department of Biological EngineeringPublisher
Massachusetts Institute of Technology