Physics-informed Deep Learning and Differentiable Mechanistic Models for Multicomponent Transport Phenomena
Author(s)
Rehman, Danyal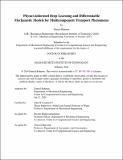
DownloadThesis PDF (20.98Mb)
Advisor
Lienhard V, John H.
Terms of use
Metadata
Show full item recordAbstract
A large subset of critical metals in today’s world occur in abundance across diverse bodies of water. For example, 83% of global lithium reserves are found in salt lakes and geothermal brines. Harvesting these metals, however, is often a separations challenge due to the presence of other solutes with similar solubility products and ionic radii. Membrane-based processes have shown great promise in performing these separations with high fractionation efficacy and energy-efficiency, motivating further study. To better optimize these systems and predict performance outside calibration limits, computational methods are essential. Extant methods leverage partial differential equation (PDE)-based continuum models that introduce a multitude of physical assumptions to improve computational tractability. These assumptions typically oversimplify the governing dynamics posing significant challenges to model generalization at new operating conditions. Moreover, these PDE-based models necessitate tedious characterization procedures that require vast amounts of experimental data for calibration, which simultaneously overconstrain regression parameters further impeding generalization performance. In this thesis, we alleviate many of these concerns through two distinct approaches: (1) the design and analysis of new differentiable mechanistic models for multicomponent membrane transport; (2) the development and validation of physics-informed deep learning surrogate models for transmembrane transport phenomena. In the former, we relax many of the assumptions present in traditional models, while introducing more physically-representative relationships like pore size distributions, fictitious image charges, etc. In addition, we combine these efforts with Monte Carlo simulations to rigorously quantify model uncertainty, engendering models that are more accurate than the state-of-the-art. Lastly, we pose new hybrid global-local optimization strategies capable of reducing the amount of data required for characterization by over 80%. In the second approach, we develop neural differential equation (NDE)-based models capable of learning smooth ion rejection profiles typical of membrane-based systems. These methods also leverage the attention mechanism, akin to that observed in language models, to elucidate paired transport relationships that dictate transmembrane ion transport. Further, we adopt transfer learning strategies conditioned on conventional PDE-based models in conjunction with integrated inductive biases like charge conservation, substantially narrowing the scope of feasible solutions. Our proposed deep learning frameworks achieve superior generalization performance over state-of-the-art mechanistic models, demonstrating the value of deep learning alternatives to conventional methods.
Date issued
2024-02Department
Massachusetts Institute of Technology. Department of Mechanical EngineeringPublisher
Massachusetts Institute of Technology