Bayesian Inference and Experimental Design of Combustion Kinetic Models
Author(s)
Chen, Huaibo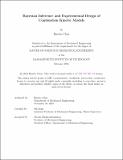
DownloadThesis PDF (8.479Mb)
Advisor
Deng, Sili
Terms of use
Metadata
Show full item recordAbstract
In combustion kinetic model calibration, researchers usually use experimental data to reduce the uncertainty of kinetic parameters, and Bayesian inference is the most common approach to do inverse calibration. This thesis explores two interconnected aspects of Bayesian approaches in the context of combustion kinetics models: how to utilize high-resolution species profiles in Bayesian inference and how to identify the most informative experimental conditions to collect data. In the first part, we investigated the impact of the effective independent-data number and target selection on Bayesian inference of kinetic parameters using species time-histories obtained from shock tube experiments. Neural networks serve as response surfaces. Maximum a posteriori estimation and Markov chain Monte Carlo sampling are employed to determine optimal parameters as well as their uncertainty. Three optimization strategies are employed: utilizing the entire species time-history curve with effective independent-data numbers of 1 (C-1) and 160 (C-160), and using only the last point of each curve (LastP). All three improved models fit experimental data better. Comparing C-1 with C-160 reveals that increasing the number of targets improves prediction accuracy but may lead to overtuning. Comparing C-1 with LastP, LastP exhibits comparable or slightly better agreement with measurements, suggesting that focusing on critical points is effective for point estimation. However, C-1 shows different posterior uncertainty from LastP in both parameters and predictions, despite their similarity in the point estimation.
Experimental data obtained at different experimental conditions (e.g., pressure, temperature, equivalence ratio, etc.) is not equally informative when it is used to calibrate kinetic parameters. Thus, experimental design becomes an important topic in combustion kinetics, where the most informative condition can be identified by algorithms. In the second part, we propose an efficient Bayesian experimental design algorithm that integrates Laplacian approximation-based experimental design with gradient-based design optimization, employing sophisticated neural network response surfaces for mapping kinetic parameters to target prediction at a wide range of thermodynamic conditions. The algorithm demonstrates efficiency and robustness against local maxima. Additionally, to meet various needs in kinetic experiments, we develop various experimental design targets based on the posterior covariance matrix, including model-oriented, parameter-oriented, target-oriented, and parallel experimental design. The proposed method, utilizing a full posterior covariance matrix without fixing any parameter of insensitive reactions, achieves significant acceleration compared to previous methods, demonstrating effectiveness in reducing parameter and target uncertainty as well as designing multiple experiments simultaneously.
Date issued
2024-02Department
Massachusetts Institute of Technology. Department of Mechanical EngineeringPublisher
Massachusetts Institute of Technology