To attract or to oscillate: Validating dynamics with behavior
Author(s)
Murray, Keith T.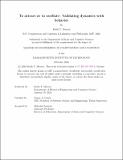
DownloadThesis PDF (17.54Mb)
Advisor
Lynch, Nancy A.
Terms of use
Metadata
Show full item recordAbstract
In recent years, the `computation-through-dynamics' framework has gained traction within the neuroscience community as a means of describing how neurological processes implement behavioral computations. The framework argues that computations in neural systems are best explained through dynamical systems in which behaviorally-relevant variables are represented and manipulated via dynamical phenomena. While a variety of previous works have demonstrated the framework's productivity, there are a number of challenges surrounding its efficacy. In this thesis, we identify and address two challenges concerning the existence of multiple dynamical systems which perform the same computation.
We show that a continuous-time recurrent neural network (CT-RNN) can implement two distinct dynamical systems, termed the ``attractive mechanism'' and the ``oscillatory mechanism'', to compute a novel modular arithmetic task inspired by the card game SET. The attractive mechanism computes modular arithmetic through traversing a lattice of fixed-point attractors. The oscillatory mechanism computes modular arithmetic through phase-shifts on a limit cycle. The existence of these two dynamical mechanisms raises two challenges for the `computation-through-dynamics' framework:
1. How can computationally similar, yet dynamically distinct systems be experimentally identified?
2. What criteria determine the implementation of one dynamical system versus another?
We address these questions by advocating for the use of behavioral phenomena. Through two experiments, we show how our dynamical mechanisms produce distinct psychometric curves when classifying ambiguous stimuli and generalize to unseen stimuli at different rates when trained on partial datasets. We further argue how these behavioral phenomena can serve as ecological criteria in determining the implementation of a mechanism. These results underscore the utility of behavior in the `computation-through-dynamics' framework.
We conclude this thesis by formulating levels of abstraction for the `computation-through-dynamics' framework, termed `levels of neural computation'. Levels of abstraction were critically important in establishing the efficacy of digital computation; therefore, we speculate that the `levels of neural computation' will further advance the efficacy of the framework. These levels argue for interpreting dynamical systems as implementations for more abstract `geometric representations and manipulations' that effectively serve as neural algorithms.
Date issued
2024-02Department
Massachusetts Institute of Technology. Department of Brain and Cognitive SciencesPublisher
Massachusetts Institute of Technology