Task-optimized models of human hearing link perception and neural coding
Author(s)
Saddler, Mark R.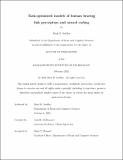
DownloadThesis PDF (28.10Mb)
Advisor
McDermott, Josh H.
Terms of use
Metadata
Show full item recordAbstract
Hearing allows organisms to derive information about the world from sound. The ears convert pressure waves into patterns of neural activity which the brain can use to make powerful inferences about the source. While extensive modeling efforts in the past few decades have resulted in well-established computational descriptions of peripheral auditory coding, comparatively less is known about how this neural code supports complex auditory behavior. Humans with normal hearing are remarkably adept at recognizing and localizing sounds in noisy environments with multiple competing sources. However, these abilities are fragile and are greatly compromised in listeners with hearing loss or cochlear implants, often leading to frustration and social isolation. Current assistive devices largely fail to aid impaired listeners in noisy environments, and the development of more effective devices is currently limited by an incomplete understanding of which features of neural coding underlie perception. This thesis develops computational models for explicitly linking specific features of peripheral auditory processing and perception. In a series of three studies, we optimized deep artificial neural network models to perform real-world hearing tasks using simulated auditory nerve input. The first study outlined a framework for optimizing models under different conditions to test how perception is constrained by our ears and acoustic environment in the domain of pitch perception. The second study extended the framework to examine the widely debated perceptual role of auditory nerve spike timing in hearing more broadly. The third study explored a practical application of task-optimized models by leveraging intermediate model representations as a perceptual metric for speech enhancement. Collectively, the results link aspects of hearing to environmental and neural coding constraints, illustrating the utility of artificial networks to reveal underpinnings of behavior.
Date issued
2024-02Department
Massachusetts Institute of Technology. Department of Brain and Cognitive SciencesPublisher
Massachusetts Institute of Technology