City-scale Cross-view Geolocalization with Generalization to Unseen Environments
Author(s)
Downes, Lena M.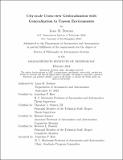
DownloadThesis PDF (13.97Mb)
Advisor
How, Jonathan P.
Steiner III, Theodore J.
Terms of use
Metadata
Show full item recordAbstract
Cross-view geolocalization, a supplement or replacement for GPS, provides an estimate of an agent's global position by matching a local ground image to an overhead satellite image. It is challenging to reliably match these two sets of images in part because they have significantly different viewpoints. Existing works have demonstrated geolocalization in constrained scenarios over small areas using panoramic cameras, yielding methods that have limited generalization to unseen environments or conditions and that do not quantify uncertainty. This thesis details Wide-Area Geolocalization (WAG) and Restricted FOV Wide-Area Geolocalization (ReWAG) that combine a neural network with a particle filter to achieve global position estimates for a moving agent in a GPS-denied environment while scaling efficiently to city-sized regions in unseen environments and working with either panoramic or non-panoramic cameras. One contribution is a trinomial loss function that enables accurate and computation-efficient localization across city-scale search areas of nearly 300 km^2 in size by improving image retrieval on the off-center image pairs that result from a coarsely discretized satellite image database. Another contribution is a computationally efficient method to incorporate pose information with input image pairs, which improves localization accuracy with non-panoramic cameras and off-center ground images. An additional contribution is the GKL uncertainty measure for localization outputs, which enables detection of particle filter false convergence through characterization of the particle distribution. The final contribution is a demonstration of ReWAG's ability to generalize across different times of day, seasons, weather, and cameras on data collected from a moving car in Cambridge, Massachusetts, as well as the public release of a challenging imagery dataset collected on this vehicle platform. WAG and ReWAG localize from over 1 km to less than 100 m of localization error while performing particle filter updates with less than 1% of the computation required for previous approaches.
Date issued
2024-02Department
Massachusetts Institute of Technology. Department of Aeronautics and AstronauticsPublisher
Massachusetts Institute of Technology