Personalized Treatment Response Prediction under Dynamic and Time-Varying Treatment Strategies for Sepsis Patients
Author(s)
Su, Megan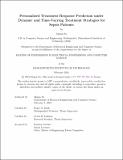
DownloadThesis PDF (1.762Mb)
Advisor
Mark, Roger G.
Lehman, Li-wei H.
Terms of use
Metadata
Show full item recordAbstract
Sepsis is a life-threatening medical emergency in which the body responds improperly to an infection, and is typically treated with intravanous fluids and vasopressors. However, administering the right balance is often difficult because adverse outcomes can be caused by both excessive and insufficient treatment. There have been many clinical trials done in the past to investigate the optimal regime for treating sepsis, however these studies have resulted in inconclusive results and often take a long time to conduct. Thus, personalized treatment response prediction under dynamic time-varying treatment strategies can be a very useful tool for clinicians when deciding what treatment strategy to administer to a patient.
This thesis builds on G-Net, a deep sequential modeling framework for g-computation that has been evaluated on response prediction under dynamic and time-varying strategies on the population level. Utilizing real-world data collected from the intensive care unit (ICU), we evaluate the performance of various deep learning implementations of G-Net on individual-level response prediction and compare their performances on prediction under the observational treatment regime. We then apply G-Net to counterfactual prediction under alternative regimes of interest and show that G-Net is able predict patient covariates and outcomes that are physiologically plausible and match clinical intuition. Our work showcases the potential of G-Net as a tool for personalized treatment response prediction to aid clinicians in determining optimal therapy for sepsis patients in the ICU.
Date issued
2024-02Department
Massachusetts Institute of Technology. Department of Electrical Engineering and Computer SciencePublisher
Massachusetts Institute of Technology