Accelerating Flow-Based Sampling for Large-𝑁 Gauge Theories
Author(s)
Zhang, Michael S.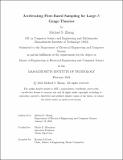
DownloadThesis PDF (603.2Kb)
Advisor
Shanahan, Phiala E.
Terms of use
Metadata
Show full item recordAbstract
Due to its consistency with numerous experimental observations, the Standard Model of particle physics is widely accepted as the best known formulation of elementary particles and their interactions. However, making experimental predictions using the Standard Model involves mathematical and computational challenges due to its complexity. Quantum chromodynamics (QCD), which can be described as an SU(3) gauge theory due to the 3 quark colors and 8 gluon types, is one sector of the Standard Model for which computing solutions is especially challenging. A natural theoretical generalization of QCD is the class of all SU(𝑁) gauge theories; these theories also provide a method for some QCD computations in the 𝑁 → ∞ limit. To study these theories numerically, approximations are calculated from configuration samples due to the mathematical complexity and lack of analytical solutions.
In this thesis, we explore asymptotically efficient flow-based sampling algorithms for the twisted Eguchi-Kawai (TEK) model, a method for analyzing large-𝑁 QCD numerically. We introduce an original architecture based on SU(2) matrix multiplication that allows for efficient Jacobian computation. In addition, we explore the possibility of transfer learning with respect to the number of colors 𝑁 and demonstrate that a model trained quickly on the SU(𝑁) setting also provides useful information in SU(𝑁′), 𝑁′ > 𝑁 cases.
Date issued
2024-02Department
Massachusetts Institute of Technology. Department of Electrical Engineering and Computer SciencePublisher
Massachusetts Institute of Technology