Designing Macromolecules using Machine Learning and Simulations
Author(s)
Mohapatra, Somesh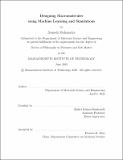
DownloadThesis PDF (37.69Mb)
Advisor
Gómez-Bombarelli, Rafael
Terms of use
Metadata
Show full item recordAbstract
The near-infinite number of possible macromolecules, arising from the combinations of monomers, linkages, and their topological arrangement, contributes to the ubiquity and indispensability of macromolecules. However, such chemical diversity hinders the development of general computational approaches that can be applied to macromolecules. The challenges around representing, comparing and learning over macromolecules are manifold. Current representations provide limited coverage of chemical space, and require significant customization to include non-natural monomers and non-linear topologies. Similarity computation methods are limited to biological macromolecules, incorporate evolutionary bias in scoring, and generally do not extend to unnatural monomers or non-linear topologies. Machine learning models are restricted by descriptors with limited representation capacity. To address these challenges, we developed chemistry-informed representations for the individual monomer unit and the complete macromolecule to capture both the local chemistry and global topology. Chemical similarity computation methods were developed to compare two or more macromolecules, irrespective of monomer chemistry and topology. A wide variety of unsupervised and supervised machine learning methods, selected according to the macromolecule type, data set size, and task, were used to identify patterns in unlabeled data sets, and map macromolecules to properties in labeled data sets, respectively. Using attribution analysis over the pre-trained models, we interpreted the decision-making process of the models. We applied these tools for de novo design, virtual screening, and in silico optimization of macromolecules, mostly followed by experimental validation of predictions, for applications ranging from peptides and glycans, to electrolytes and thermosets.
Date issued
2022-05Department
Massachusetts Institute of Technology. Department of Materials Science and EngineeringPublisher
Massachusetts Institute of Technology