Likelihood-Free Hypothesis Testing and Applications of the Energy Distance
Author(s)
Gerber, Patrik Róbert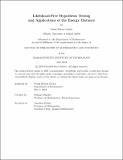
DownloadThesis PDF (2.918Mb)
Advisor
Rigollet, Philippe
Terms of use
Metadata
Show full item recordAbstract
This thesis studies questions in nonparametric testing and estimation that are inspired by machine learning. One of the main problems of our interest is likelihood-free hypothesis testing: given three samples X, Y and Z with sample sizes n, n and m respectively, one must decide whether the distribution of Z is closer to that of X or that of Y . We fully characterize the problem’s sample complexity for multiple distribution classes and with high probability. We uncover connections to two-sample, goodness-of-fit and robust testing, and show the existence of a trade-off of the form mn ≍ k/ε^4, where k is an appropriate notion
of complexity and ε is the total variation separation between the distributions of X and Y . We generalize our problem to allow Z to come from a mixture of the distributions of X and Y , and propose a kernel-based test for its solution, and also verify the existence of a trade-off between m and n on experimental data from particle physics. In addition, we demonstrate that the family of “classifier accuracy” tests are not only popular in practice but also provably near-optimal, recovering and simplifying a multitude of classical and recent results. Finally, we study affine classifiers as a tool for estimation and testing, with the key technical tool being a connection to the energy distance. In particular, we propose a density estimation routine based on minimizing the generalized energy distance, targeting smooth densities and Gaussian mixtures. We interpret our results in terms of half-space separability over these classes, and derive analogous results for discrete distributions. As a consequence we deduce that any two discrete distributions are well-separated by a half-space, provided their support is embedded as a packing of a high-dimensional unit ball. We also scrutinize two recent applications of the energy distance in the two-sample testing literature.
Date issued
2024-05Department
Massachusetts Institute of Technology. Department of MathematicsPublisher
Massachusetts Institute of Technology