Reinforcement Learning for Cybersecurity Risk Assessment of Advanced Air Mobility Systems
Author(s)
Pieper, Brenton A.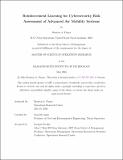
DownloadThesis PDF (3.454Mb)
Advisor
Amin, Saurabh
Terms of use
Metadata
Show full item recordAbstract
Modern AI/ML tools have significant potential to accelerate the development of Advanced Air Mobility (AAM) systems that use unmanned aerial systems for providing mobility services. The efficacy of these systems relies on highly granular, reliable, and trustworthy sensor data. This thesis is motivated by the need to assess safety risks due to cyber vulnerabilities in the surveillance components of AAM systems such as Automatic Dependent Surveillance-Broadcast (ADS-B) and the Airborne Collision Avoidance System (ACAS). We focus on spoofing attacks targeted at specific AAM agents and develop a computational approach to evaluate the impact of such attacks on the performance of cooperative agents modeled in a Multi-Agent Reinforcement Learning (MARL) framework. Our threat model is particularly suited for quantifying the safety risks of nominally trained MARL algorithms under attacks by an adversary capable of compromising observational data of a single target agent. In contrast to prior work in Adversarial RL, our approach to creating adversarial perturbations does not require access to learning and control mechanisms internal to the compromised agent. We show how realistic spoofing attacks can be successfully constructed using a simulated MARL-based AAM system, called AAM-Gym. We then conduct a safety risk analysis of such attacks using commonly accepted aviation safety metrics. Specifically, we find that safety compliance decreases across multiple aircraft densities under a spoofing attack to a single agent, owing to higher risk of Near Mid-Air Collision (NMAC). Finally, to understand possible algorithmic defenses, we take inspiration from Safe RL and show how AAM agents can be made more robust, and hence more safety compliant, to observational spoofing by using a minimax training criterion. Our work highlights the need to rigorously study the safety risks of AAM systems under realistic cyber threat models. Our findings can benefit efforts to develop practical defense techniques, such as signal validation and filtering, to detect the presence of adversarial perturbations, and control algorithms to adapt and respond to safety compromises in a timely manner.
Date issued
2024-05Department
Massachusetts Institute of Technology. Operations Research Center; Sloan School of ManagementPublisher
Massachusetts Institute of Technology