Human-Inspired Methods for Extending Advances in Computer Vision to Data- and Compute-Constrained Environments
Author(s)
Brandt, Laura E.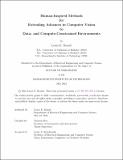
DownloadThesis PDF (4.555Mb)
Advisor
Roy, Nicholas
Terms of use
Metadata
Show full item recordAbstract
Recent developments in computer vision have often relied on access to big data, powerful compute, or both. City-based systems, such as self-driving cars and airport checkpoints, have benefited greatly from these advances, so much so that automated cars and security checks are beginning see true deployment in modern society. In contrast, robots and autonomous systems in data- and compute- constrained environments, like remote wilderness regions or off-Earth, are still relying on pre-deep learning era computer vision algorithms. Robots in the most challenging of environments - and, correspondingly, the environments most crucial to automate - have been left behind by modern computer vision.
In this dissertation, I discuss several human-inspired methods that I have studied during my time at MIT, with the goal of closing the gap between modern computer vision and data- and compute- constrained environments. I explore methods for collecting good - but small - datasets, upsampling lazily-computed visual estimates to improve their quality, and identifying which image samples (and sample regions) a learned model is `uncertain' about. Finally, I share sketches of several human-inspired paradigms for leveraging these new tools to make vision models more efficient and generalisable, which I hope can serve as a starting point for future efforts to close the gap and bring modern vision to deployment in data- and compute- constrained environments.
Date issued
2024-05Department
Massachusetts Institute of Technology. Department of Electrical Engineering and Computer SciencePublisher
Massachusetts Institute of Technology