MeMo: Meaningful, Modular Controllers via Noise Injection
Author(s)
Tjandrasuwita, Megan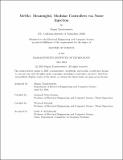
DownloadThesis PDF (6.690Mb)
Advisor
Solar-Lezama, Armando
Matusik, Wojciech
Terms of use
Metadata
Show full item recordAbstract
Robots are often built from standardized assemblies, (e.g. arms, legs, or fingers), but each robot must be trained from scratch to control all the actuators of all the parts together. In this paper we demonstrate a new approach that takes a single robot and its controller as input and produces a set of modular controllers for each of these assemblies such that when a new robot is built from the same parts, its control can be quickly learned by reusing the modular controllers. We achieve this with a framework called MeMo which learns (Me)aningful, (Mo)dular controllers. Specifically, we propose a novel modularity objective to learn an appropriate division of labor among the modules. We demonstrate that this objective can be optimized simultaneously with standard behavior cloning loss via noise injection. We benchmark our framework in locomotion and grasping environments on simple to complex robot morphology transfer. We also show that the modules help in task transfer. On both structure and task transfer, MeMo achieves improved training efficiency to graph neural network and Transformer baselines.
Date issued
2024-05Department
Massachusetts Institute of Technology. Department of Electrical Engineering and Computer SciencePublisher
Massachusetts Institute of Technology