Offline Reward Learning from Human Demonstrations and Feedback: A Linear Programming Approach
Author(s)
Kim, Kihyun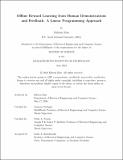
DownloadThesis PDF (545.9Kb)
Advisor
Ozdaglar, Asuman
Parrilo, Pablo A.
Terms of use
Metadata
Show full item recordAbstract
In many complex sequential decision-making tasks, there is often no known explicit reward function, and the only information available is human demonstrations and feedback data. To infer and shape the underlying reward function from this data, two key methodologies have emerged: inverse reinforcement learning (IRL) and reinforcement learning from human feedback (RLHF). Despite the successful application of these reward learning techniques across a wide range of tasks, a significant gap between theory and practice persists. This work aims to bridge this gap by introducing a novel linear programming (LP) framework tailored for offline IRL and RLHF. Most previous work in reward learning has employed the maximum likelihood estimation (MLE) approach, relying on prior knowledge or assumptions about decision or preference models. However, such dependencies can lead to robustness issues, particularly when there is a mismatch between the presupposed models and actual human behavior. In response to these challenges, recent research has shifted toward recovering a feasible reward set, a general set of rewards where the expert policy is optimal. In line with this evolving perspective, we focus on estimating the feasible reward set in an offline context. Utilizing pre-collected trajectories without online exploration, our framework estimates a feasible reward set from the primal-dual optimality conditions of a suitably designed LP, and offers an optimality guarantee with provable sample efficiency. One notable feature of our LP framework is the convexity of the resulting solution set, which facilitates the alignment of reward functions with human feedback, such as pairwise trajectory comparison data, while maintaining computational tractability and sample efficiency. Through analytical examples and numerical experiments, we demonstrate that our framework has the potential to outperform the conventional MLE approach.
Date issued
2024-05Department
Massachusetts Institute of Technology. Department of Electrical Engineering and Computer SciencePublisher
Massachusetts Institute of Technology