Learning the language of biomolecular interactions
Author(s)
Sledzieski, Samuel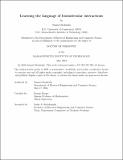
DownloadThesis PDF (24.47Mb)
Advisor
Berger, Bonnie
Terms of use
Metadata
Show full item recordAbstract
Proteins are the primary functional unit of the cell, and their interactions drive cellular function. Interactions between proteins are responsible for a wide variety of functions raning from catalytic activity to cellular transport and signaling, and interactions between small molecules and proteins are the foundation of many therapeutics. However, the experimental determination of these interactions is expensive and relatively slow, limiting the ability to model interactions at genome scale. It is therefore critical to develop computational approaches for modeling these interactions. Unsupervised language models trained on amino acid sequences, namely protein language models, learn patterns in sequence evolution that encode protein structure and function. These protein language models are thus a powerful tool for extracting features of proteins, enabling the adoption of lightweight downstream models. Here, we present novel machine learning techniques for adapting protein language modeling to the prediction of protein interactions at scale, enabling de novo interaction network inference and large-scale drug compound screening. We show that these methods achieve state-of-the-art performance, and allow us to discover new biology and therapeutic candidates. In addition, we introduce methods for efficient training and adaptation of these models, and outline several applications which take advantage of the scale enabled by lightweight models. As a whole, this thesis demonstrates how computational advances in language modeling and the massive growth of data brought about by the sequencing revolution can be leveraged to tackle the genotype-to-phenotype challenge in biology, and lays the groundwork for more widespread adoption of these techniques for proteomic modeling.
Date issued
2024-05Department
Massachusetts Institute of Technology. Department of Electrical Engineering and Computer SciencePublisher
Massachusetts Institute of Technology