Developing a Machine Learning Based Automated Screening Tool to Diagnose Silent Heart Attacks in Resource-Constrained Settings
Author(s)
Real, Karyn N.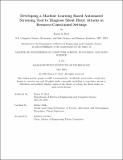
DownloadThesis PDF (4.043Mb)
Advisor
Duŕo, Esther
Terms of use
Metadata
Show full item recordAbstract
Aging populations worldwide pose significant financial and social challenges for low- and middle-income countries, particularly in supporting elderly individuals with chronic illnesses. These challenges are exacerbated by a shortage of high-fidelity diagnostic technology. While point-of-care tests offer a low-cost and mobile solution to limited diagnostic access, they lack the precision of more expensive tests and specialized medical expertise. This thesis develops a supervised machine learning-based diagnostic tool for silent heart attacks using both point-of-care and gold standard data from elders in Tamil Nadu, India, as part of a proposed solution to bridge the diagnostic gap. The research explores whether point-of-care data can be used to reliably identify risk, transforming low-cost inputs into predictions with signal. We also investigate how to operationalize these predictions in a referral pipeline. The results demonstrate that single-lead ECG inputs can effectively detect signals indicative of silent heart attacks. Based on model predictions and a cost-benefit analysis, we suggest risk score thresholds for classifying high-risk individuals for whom it is cost-effective to refer to escalated care. Additionally, integrating single-lead and 12-lead ECG data enhances diagnostic accuracy and supports the development of an operational referral pipeline.
Date issued
2024-05Department
Massachusetts Institute of Technology. Department of Electrical Engineering and Computer SciencePublisher
Massachusetts Institute of Technology