Multi-Modal Protein Function Prediction using a Joint Embedding Space from Two Graph Neural Networks
Author(s)
Tysinger, Emma P.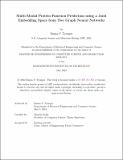
DownloadThesis PDF (13.29Mb)
Advisor
Kellis, Manolis
Terms of use
Metadata
Show full item recordAbstract
In bioinformatics and proteomics, determining protein functions experimentally is expensive and slow. There’s a growing need for precise and quick computational prediction methods, filling the gap between sequence discovery and functional understanding. Over recent years there has been an influx of deep-learning protein folding algorithms used for predicting function by transfer learning. Protein function is only partially captured by each of a large number of modalities including structure, however, in isolation they only give us a partial understanding of function. Uniting these is an important step to understanding function more holistically. We present a multi-modal framework using two graph neural networks to infer a joint embedding space that captures many properties of a protein including structure, disease associations, drug interactions, protein interactions, biological processes and more. We evaluate the embedding space on downstream prediction tasks including enzyme commission (EC) numbers and gene ontology (GO) terms. Experimental results on protein function prediction, as well as a qualitative visual analysis of the protein embedding space show that our framework is able to successfully capture both structure and biomedical context of proteins, and outperforms structure-only based encoders.
Date issued
2024-05Department
Massachusetts Institute of Technology. Department of Electrical Engineering and Computer SciencePublisher
Massachusetts Institute of Technology