Leveraging Multi-Stage Machine Learning Pipelines for Extracting Structured Key-Value Pairs from Documents
Author(s)
Pyo, Bryan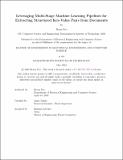
DownloadThesis PDF (2.043Mb)
Advisor
Gupta, Amar
Terms of use
Metadata
Show full item recordAbstract
In the rapidly growing field of information extraction, the ability to automatically and accurately extract structured data from sources has grown in importance across several industries. This need has arisen largely due to the vast quantity of data that is currently available and still being actively collected by these industries for various purposes. In a world where data has grown greatly in quantity and importance, the ability to parse this data into usable information has grown to become an even more essential endeavor. Although information extraction has traditionally been a relatively labor-intensive task, with the rising sophistication and applicability of machine learning and computer-aided document analysis, automatic and more generalized methods of extracting relevant data from documents have become a major focus of research. This thesis discusses several pipelines that have been developed to extract data in the form of key-value pairs from specification sheets describing mechanical parts achieving accuracies ranging from 80% to 100% depending on the pipeline and the target documents and key-value pairs.
Date issued
2024-05Department
Massachusetts Institute of Technology. Department of Electrical Engineering and Computer SciencePublisher
Massachusetts Institute of Technology