Rethinking the Evaluation of Compositional Reasoning for Modern VLMs
Author(s)
Huang, Irene Y.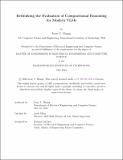
DownloadThesis PDF (8.565Mb)
Advisor
Oliva, Aude
Terms of use
Metadata
Show full item recordAbstract
Recent advancements in modern Vision-Language Models (VLMs), comprising a visual encoder coupled with a Large Language Model (LLM) decoder, have demonstrated remarkable proficiency in Compositional Reasoning (CR). CR entails grasping the significance of attributes, relations, and word order. This prompts a crucial question: have VLMs effectively tackled the CR challenge? Our conjecture suggests that existing CR benchmarks may not adequately push the boundaries of modern VLMs due to their reliance on a negative text generation pipeline. Consequently, the negatives produced often deviate either as outliers from the natural language distribution learned by VLMs’ LLM decoders or as improbable within the corresponding image context. To redress these limitations, we propose a novel pipeline integrating GPT-4V alongside a suite of contemporary open-source VLMs. Through the application of in-context-learning and prompt engineering methodologies, our pipeline autonomously generates, evaluates, and selects challenging compositional reasoning questions, to establish a robust CR benchmark, also subsequently validated manually. The meticulously curated dataset evinces a noteworthy, up to 45%, decrease in CR performance compared to preceding benchmarks, thereby reinstating the CR challenge even for state-of-the-art VLMs.
Date issued
2024-05Department
Massachusetts Institute of Technology. Department of Electrical Engineering and Computer SciencePublisher
Massachusetts Institute of Technology