NeuralMOVES: Extracting and Learning Surrogates for Diverse Vehicle Emission Models
Author(s)
Ramirez Sanchez, Edgar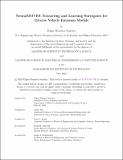
DownloadThesis PDF (6.276Mb)
Advisor
Wu, Cathy
Donti, Priya L.
Terms of use
Metadata
Show full item recordAbstract
Technological advancements and interventions in the transportation sector play a crucial role in addressing climate change, given its major contribution to greenhouse gas emissions. The industry actively explores electrification, automation, and Intelligent Infrastructure to mitigate emissions. However, the successful design and implementation of these solutions require accurate and representative emission models. The Motor Vehicle Emission Simulation (MOVES) serves as the gold standard emission software provided by the Environmental Protection Agency (EPA). Despite its prominence, using MOVES faces challenges, including a steep learning curve and technical complexities. This makes it cumbersome for macroscopic analysis and unsuitable for microscopic analyses like eco-driving, which demands emissions estimation for individual steps. To address these issues, we present a comprehensive family of high-performance and lightweight CO₂ emission models devised through reverse engineering MOVES and surrogate learning. Our models show a promising 6% end-to-end error relative to MOVES, exhibit significant differences from alternative reduced-order models, and offer improved precision. The implications of our work are twofold: our models simplify GHG emission evaluation in transportation-related analyses by providing a faster, programmatic alternative to MOVES and improve control-based approaches by offering microscopic and environment feature-rich models compared to alternative models.
Date issued
2024-05Department
Massachusetts Institute of Technology. Department of Electrical Engineering and Computer Science; Massachusetts Institute of Technology. Institute for Data, Systems, and Society; Technology and Policy ProgramPublisher
Massachusetts Institute of Technology