Uncertainty & robustness for single-cell studies
Author(s)
Shiffman, Miriam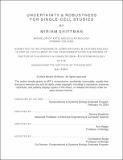
DownloadThesis PDF (110.0Mb)
Advisor
Broderick, Tamara
Regev, Aviv
Terms of use
Metadata
Show full item recordAbstract
The advent of new technologies capable of measuring molecular profiles at single-cell granularity, across thousands or millions of cells, offers unprecedented insight into the form, function, and circuitry of biological systems. At the same time, these technologies present particular statistical and computational challenges, including noise, sparsity, technical and biological variability, and multilevel sampling regimes. To distill relevant signal from biological phenomena, then, analyses must combine information in a careful and coherent way across cells. In light of these complexities, it is prudent that single-cell analyses incorporate notions of uncertainty and robustness to guide their interpretation and inform future decision making.
This thesis makes two main advances in facilitating coherent, actionable quantification of uncertainty and robustness for single-cell studies. First, we provide a framework for generalizability of differential expression analysis that—unlike common statistical tools (significance, power, standard error)—does not rely on the assumption that the sample in hand is independent and identically distributed as future samples. Instead, we posit an alternate (complementary) lens on generalizability: could dropping a very small fraction of cells meaningfully alter the basic conclusions of differential expression? We develop an accurate and efficient approximation to estimate this dropping-data robustness metric for the key outcomes of differential expression, for independent observation and pseudobulk analyses. Broadening these gene-level results to a high-level, biologically meaningful summary, we overcome the inherently non-differentiable and combinatorial nature of gene set enrichment analysis to provide an additional approximation for the dropping-data robustness of top gene sets. Applied to public single-cell RNA-seq data of healthy and diseased cells, our metric identifies widespread nonrobustness across genes that extends to high-level nonrobustness of top gene sets. The second part of this thesis provides a full Bayesian framework for reconstructing probabilistic trees of cellular differentiation from single-cell profiles. Namely, motivated by the biology of differentiation and confronted with a lack of existing hierarchical models, we develop a new family of probabilistic trees where data is generated continuously along branches (and latent cell state evolves smoothly over the tree). We also develop two approaches, focusing on gene-level or cell-level variability, to model measurement noise arising from single-cell RNA-sequencing. In tandem, we construct a novel Markov chain Monte Carlo sampler over trees, including message passing with variable augmentation to accelerate inference. These techniques recover latent trajectories from simulated single-cell transcriptomes, and make progress toward inferring trajectories, with calibrated uncertainties, from real transcriptomes.
I close by reflecting on common themes relevant to uncertainty and robustness for single-cell studies, including interplay between the continuous and the discrete, the challenge of summarization, the importance of cyclical model criticism, and a possible way forward through differentiable and probabilistic programming.
Date issued
2024-05Department
Massachusetts Institute of Technology. Computational and Systems Biology ProgramPublisher
Massachusetts Institute of Technology