Multi-Agent Reinforcement Learning for Autonomous Robotics
Author(s)
Vincent, Caroline R.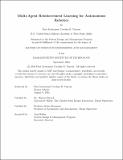
DownloadThesis PDF (2.359Mb)
Advisor
Karaman, Sertac
Ricard, Michael
Terms of use
Metadata
Show full item recordAbstract
Technological advancements in autonomous robotics, including autonomous vehicles, have created new opportunities for innovative solutions to many everyday challenges. The impact of integrating robotic agents into real-world applications may be significantly enhanced by leveraging advancements in multi-agent autonomous systems. However, the coordination required in multi-agent systems demands complex motion planning to deconflict actions and prevent collisions of vehicles moving at increasingly high speeds. This thesis explores the application of multi-agent reinforcement learning (MARL) to autonomous robotics by teaching a central controller to navigate multiple agents across various environments without collisions. The simulated scenarios range from simple, obstacle-free environments to complex environments with obstacles configured to form narrow passageways or represent other complexities in dense urban environments. The findings demonstrate the potential of MARL to achieve high accuracy in navigating these different environments, highlighting the method's flexibility and adaptability across diverse settings and the resulting implications for applying MARL to real-world scenarios.
Date issued
2024-09Department
System Design and Management Program.Publisher
Massachusetts Institute of Technology