Comparison of Machine Learning-Based Methods for Narrowband Blind Adaptive Beamforming
Author(s)
Shonkwiler, Lara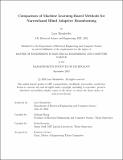
DownloadThesis PDF (3.954Mb)
Advisor
Zheng, Lizhong
Forsythe, Keith
Terms of use
Metadata
Show full item recordAbstract
There are many different approaches to beamforming and interferer cancellation. The earliest methods of beamforming assumed prior knowledge of the receive array geometry and of the incoming signal directions. This information is normally found via array calibration. Blind source separation methods do not require this information and therefore are more robust to array calibration errors. Traditional blind source separation methods generally leverage some intrinsic characteristic of the signal, such as constant envelope properties or second or higher order statistics. Traditional blind source separation methods such as CMA, SOBI, JADE, and FastICA tend to be highly effective at beamforming datasets with moderate to large sample supports, but they do not perform well when they only have access to a limited number of data samples. They also bear the disadvantage that the appropriate algorithm must be selected based on the properties of the expected signal. Machine learningbased methods are of interest because they show promise in low sample support regimes, and because they offer the possibility of a ‘one size fits all’ solution that can adaptively recognize and exploit different signal features. This thesis describes the performance of two machine learning-informed beamforming methods — Classification-Based Transfer Learning (CBTL) [1] and Denoising-Based Transfer Learning (DBTL). CBTL and DBTL are evaluated with respect to each other and with respect to traditional blind beamforming methods across a variety of signal detection environments, and are found to offer superior or equivalent performance in a majority of environments.
Date issued
2024-09Department
Massachusetts Institute of Technology. Department of Electrical Engineering and Computer SciencePublisher
Massachusetts Institute of Technology