Physics-Informed Deep Learning for Plasma Etch Optimization
Author(s)
Dighamber, Mohit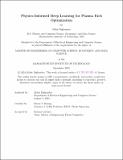
DownloadThesis PDF (12.00Mb)
Advisor
Boning, Duane S.
Terms of use
Metadata
Show full item recordAbstract
Modeling the plasma etch process is highly valuable in the field of semiconductor manufacturing. This intricate process relies on the execution of numerous individual processes, numbering in the hundreds to thousands. The application of artificial intelligence (AI) and machine learning (ML) techniques in optimizing these steps presents a revolutionary prospect for the industry. In this thesis, we share our findings for plasma etch recipe optimization using AI and ML. We present a series of plasma etch datasets generated from ViennaPS, as well as a new data augmentation algorithm for generating a uniform spatial and temporal distribution of data points. Our results on this dataset demonstrate improved model generalization using physically-informed neural networks (PINNs) with a level-set based loss. We highlight performance metrics for Soft-Adapt learned physics loss weights, as well as statically chosen weights. Future work includes utilizing the PINN model in a Bayesian framework to facilitate recipe optimization for the desired etch profile.
Date issued
2024-09Department
Massachusetts Institute of Technology. Department of Electrical Engineering and Computer SciencePublisher
Massachusetts Institute of Technology