From Words to Worlds: Bridging Language and Thought
Author(s)
Wong, Lionel Catherine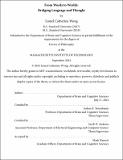
DownloadThesis PDF (16.69Mb)
Advisor
Tenenbaum, Joshua B.
Andreas, Jacob D.
Terms of use
Metadata
Show full item recordAbstract
What do we understand when we understand language? Human language offers a broad window into the landscape of our thoughts. We talk about what we see, believe, and imagine, posing questions and communicating our plans. Language, in turn, stocks our mental inventories with new concepts and theories, communicating ideas that we might not otherwise have discovered by thinking on our own even over the course of a lifetime. How do we make meaning from language, and how, in turn, does the meaning we construct from language draw on the other resources and capacities of human thought, from perception, to mental simulation and decision making? This thesis proposes a computational framework for modeling language-informed thinking, organized into two parts. In the first, I overview the overarching framework that makes up the backbone of this thesis, Rational Meaning Construction, which proposes how natural language can construct arbitrary expressions in a flexible, symbolic, and probabilistic language of thought that supports general inferences. I present examples and experiments demonstrating the range of this theory, modeling how concrete propositions and questions in language can update and query beliefs about many different domains of knowledge. In the second section, I turn to language that communicates more abstract conceptual knowledge – generic background concepts and theories that we can learn from language, and which give us building blocks for representing more concrete beliefs. I present three models that build on the basic premises of Rational Meaning Construction to learn new lexical concepts and theories from language. The first models how we can learn new theories from generic sentences that explicitly communicate or implicitly presuppose abstract knowledge. The second elaborates on this model to also incorporate environmental feedback alongside information from language. The third suggests how we can learn the meanings of new words from scratch, with very little linguistic data, using principles of both representational and communicative efficiency to guide learning. I conclude by discussing a open questions that this thesis raises about how we learn and understand language, and outline future directions that might make progress on answering them.
Date issued
2024-09Department
Massachusetts Institute of Technology. Department of Brain and Cognitive SciencesPublisher
Massachusetts Institute of Technology