Comparing Parameter Efficient Finetuning Techniques (PEFT) using Datamodels
Author(s)
Chamdal, Harshal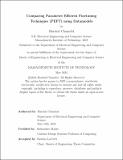
DownloadThesis PDF (669.0Kb)
Advisor
Mądry, Aleksander
Terms of use
Metadata
Show full item recordAbstract
Advances in machine learning, particularly through algorithmic innovations and large datasets, have led to models with hundreds of billions of parameters. Deploying these models is challenging and costly, especially due to the extensive finetuning required. Parameter-efficient finetuning techniques (PEFT) have been proposed to address this issue by significantly reducing the number of trainable parameters, achieving comparable results to full-parameter finetuning. Despite widespread adoption, PEFT methods are often used interchangeably without considering their qualitative differences and performance under various data distributions. This thesis extensively compares three PEFT methods: LoRA, BitFit, and (IA)³, using the ModelDiff framework to identify and apply data interventions. Our analysis reveals that the performance of these methods varies widely with different interventions, with BitFit showing the most variance, while LoRA and (IA)³ demonstrate greater resilience. This study informs the selection and optimization of PEFT techniques based on specific NLP task requirements, balancing performance, computational efficiency, and robustness to text variations.
Date issued
2024-05Department
Massachusetts Institute of Technology. Department of Electrical Engineering and Computer SciencePublisher
Massachusetts Institute of Technology