Developing frameworks for an equitable future: from building decarbonization to generative modeling.
Author(s)
De Simone, Zoe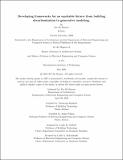
DownloadThesis PDF (5.226Mb)
Advisor
Reinhart, Christoph
Wilson, Ashia
Terms of use
Metadata
Show full item recordAbstract
In this thesis I develop computational frameworks to understand equity under two perspectives: building decarbonization policy and generative modeling.
Part 1 - Equitable building decarbonization
Buildings significantly contribute to global carbon emissions, necessitating urgent decarbonization to meet 2050 climate targets. The U.S. strives for net-zero emissions by 2050, supported by federal incentives promoting building upgrades. However, financing deep retrofits for all U.S. homes exceeds available public funds. This chapter proposes a model that examines long-term carbon reduction trajectories under various incentive policies, focusing on fairness and equity. Using Oshkosh, WI, as a case study, it explores the philosophical, economic, political, and mathematical dimensions of creating just and effective decarbonization policies that ensure healthy, low-carbon homes for all.
Part 2 - Equitable diffusion models
Generative Text-to-Image (TTI) models, while capable of producing high-quality images, often replicate training data biases. Traditional fairness views in machine learning, which consider fairness as binary, are challenged. This section introduces DiffusionWorldViewer, a novel framework with a Web UI that enables users to analyze the underlying worldviews of diffusion models and edit model outputs to align with their personal fairness perspectives, thus promoting a diverse understanding of fairness in AI technologies.
Date issued
2024-05Department
Massachusetts Institute of Technology. Department of Electrical Engineering and Computer Science; Massachusetts Institute of Technology. Department of ArchitecturePublisher
Massachusetts Institute of Technology