Multifidelity Methods for Design of Transition MetalComplexes
Author(s)
Janet, Jon Paul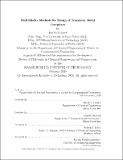
DownloadThesis PDF (25.33Mb)
Advisor
Kulik, Heather J.
Marzouk, Youssef
Terms of use
Metadata
Show full item recordAbstract
The rational design of materials with tightly controlled properties is crucial to addressing future challenges in energy, electronics and catalysis. While improvements in computing power have made simulation with density functional theory (DFT) an essential tool in screening new materials, it remains too costly to address truly high-dimensional design spaces. This problem is especially acute for open-shell transition metal (TM) complexes, which are of central importance in homogeneous catalysis and have applications in solar energy and electronics. The space of TM complexes is enormous and poorly characterized, while DFT calculations for these systems are expensive and sensitive to method choice, making it impractical to simulate large numbers of candidates indiscriminately. This makes the search for TM complexes with desired properties a formidable challenge. This thesis addresses this challenge by formulating strategies for materials design that exploit insights from data-driven surrogate models together with first-principles simulations. A framework for data-driven inference of the quantum properties of TM complexes is developed, using artificial neural networks (ANNs) and graph-based molecular representations that facilitate rapid screening while retaining physical meaning such that chemical insights can be extracted. Multiple sources of uncertainty that would limit the application of these methods to TM complexes are addressed. Surrogate models are trained to estimate system-specific DFT uncertainty by including data from DFT calculations with different fractions of exact exchange, and a novel uncertainty metric for data-driven discovery is proposed that quantifies the ability of ANNs to generalize to unseen data based on similarity in the learned latent space. This metric is shown to offer superior performance over existing methods. The application of these methods to virtual design problems is demonstrated with two case studies: 1) identifying spin crossover complexes from a design space of thousands using an evolutionary strategy and 2) probabilistic, multiobjective optimization of redox couples over a 3 million-complex space. The utility of this surrogate-assisted approach is evident and orders-of-magnitude accelerations are obtained over screening purely with DFT. Such strategies open the door for in silico design of some of the most challenging molecular systems at a far greater scale than ever before.
Date issued
2020-02Department
Massachusetts Institute of Technology. Department of Chemical Engineering; Massachusetts Institute of Technology. Center for Computational Science and EngineeringPublisher
Massachusetts Institute of Technology