Factorization and Compositional Generalization in Diffusion Models
Author(s)
Liang, Qiyao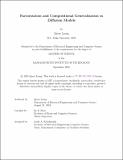
DownloadThesis PDF (24.83Mb)
Advisor
Fiete, Ila R.
Terms of use
Metadata
Show full item recordAbstract
One of the defining features of human intelligence is compositionality—the ability to generate an infinite array of complex ideas from a limited set of components. This capacity allows for the creation of novel and intricate combinations of arbitrary concepts, enabling potentially infinite expressive power from finite learning experiences. A likely prerequisite for the emergence of compositionality is the development of factorized representations of distinct features of variation in the world. However, the precise mechanisms behind the formation of these factorized representations in the human brain, and their connection to compositionality, remain unclear. Diffusion models are capable of generating photorealistic images that combine elements not co-occurring in the training set, demonstrating their ability to compositionally generalize. Yet, the underlying mechanisms of such compositionality and its acquisition through learning are still not well understood. Additionally, the relationship between forming factorized representations of distinct features and a model’s capacity for compositional generalization is not fully elucidated. In this thesis, we explore a simplified setting to investigate whether diffusion models can learn semantically meaningful and fully factorized representations of composable features. We conduct extensive controlled experiments on conditional diffusion models trained to generate various forms of 2D Gaussian data. Through preliminary investigations, we identify three distinct learning phases in the model, revealing that while overall learning rates depend on dataset density, the rates for independent generative factors do not. Moreover, our findings show that models can represent continuous features of variation with semi-continuous, factorized manifolds, resulting in superior compositionality but limited interpolation over unseen values. Based on our investigations, we propose a more data-efficient training scheme for diffusion models and suggest potential future architectures for more robust and efficient generative models.
Date issued
2024-09Department
Massachusetts Institute of Technology. Department of Electrical Engineering and Computer SciencePublisher
Massachusetts Institute of Technology