Natural Language Foundation Models in Medical Artificial Intelligence
Author(s)
Palepu, Anil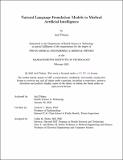
DownloadThesis PDF (41.16Mb)
Advisor
Beam, Andrew L.
Terms of use
Metadata
Show full item recordAbstract
Over the past decade, the transformative rise of deep learning, particularly large language models (LLMs), has inspired experts across diverse fields, including healthcare, to think deeply about how artificial intelligence (AI) can revolutionize their fields. In this time, general foundation models, rather than narrow and highly specialized task-specific systems, have begun to emerge as the dominant paradigm. In healthcare, AI systems are already seeing widespread implementation in a variety of real-world use cases, perhaps without adequate evaluation and validation. Indeed, their often impressive ability to process natural language, a crucial medium of knowledge and communication in medicine, suggests that many of these modern foundation models may hold immense promise in the healthcare space. However, there exists a need to better study and understand their strengths, limitations, and robustness, particularly in more realistic and clinically relevant settings.
This thesis focuses on two key classes of natural language-driven foundation models --- Contrastive Language Image Pretraining (CLIP) models, and Large Language Models (LLMs) --- and investigates how such models can encode and deliver useful clinical knowledge, for tasks like chest x-ray interpretation, differential diagnosis, history taking, and clinical management. As a whole, this thesis aims to further our collective understanding of the potential of natural language foundation models in medicine, while emphasizing the need for significant further research to address real-world challenges and understand the scopes in which such systems can be implemented safely and efficaciously.
In the first chapter, I provide an overview of some relevant background, including contrastive language-image pretrained models, large language models, and their evaluation in the medical domain.
In chapter 2, we improve the CLIP architecture for chest x-ray interpretation through a novel regularization technique applied during pre-training, and use this model for the zero-shot identification of chest x-ray findings.
In chapter 3, we examine the reliability of CLIP-style models. First, we evaluate their robustness to shortcut learning to understand the potential protective effects of text self-supervision. Next, we explore how conformal prediction can be used to control zero-shot classification performance and preempt compatible inputs for these CLIP-style models.
In chapter 4, I describe the development of Articulate Medical Intelligence Explorer (AMIE), a conversational diagnostic AI fine-tuned with simulated medical dialogue. We evaluate the diagnostic capabilities of AMIE in two randomized studies with primary care physicians; first, in challenging clinicopathological conference (CPC) cases, and then in virtual text-based objective structured clinical examinations (OSCE).
In chapter 5, we explore AMIE's management reasoning capabilities in two subspecialty domains: genetic cardiovascular disease and breast oncology. In these studies, we design domain-specific assessments for case management and compare AMIE's performance to generalists under subspecialist evaluation, as well as studying its potential assistive effect.
Date issued
2025-02Department
Harvard-MIT Program in Health Sciences and TechnologyPublisher
Massachusetts Institute of Technology