Designing Sustainable Recommender Systems
Author(s)
Huang, Lei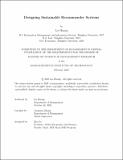
DownloadThesis PDF (1.744Mb)
Advisor
Zhang, Juanjuan
Terms of use
Metadata
Show full item recordAbstract
Recommender systems are widely deployed to serve users with content they like. However, content must be created and insufficient demand dampens a creator’s production incentive. We argue that the canonical recommender system may not be sustainable if, by promoting the content each user likes the most, it suppresses the creation incentive of the less popular but still valuable content. We propose a “sustainable recommender system” solution – subsidize creators with demand according to their “sensitivity,” which measures how easily a creator can be incentivized by demand, and their “contribution,” which measures how important a creator is to users overall. Theoretically, we prove that this algorithm maximizes long-term user utility by internalizing the externality of user choice on other users. Computationally, our main innovation is to estimate creator contribution using computer vision, where we train a deep-learning model to compute how creator distribution affects system-wide user utility. Analyzing data from a large content platform, we show that our algorithm incentivizes valuable creators and sustains long-term user experience.
Date issued
2025-02Department
Sloan School of ManagementPublisher
Massachusetts Institute of Technology