Data-Rich Personalized Causal Inference
Author(s)
Shah, Abhin .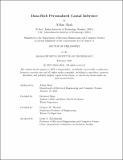
DownloadThesis PDF (4.980Mb)
Advisor
Shah, Devavrat
Wornell, Gregory W.
Terms of use
Metadata
Show full item recordAbstract
There is a growing interest in individual-level causal questions to enable personalized decision-making. For example, what happens to a particular patient’s health if we prescribe a drug to them, or what happens to a particular consumer’s behavior if we recommend a product to them? Conducting large-scale randomized experiments to answer such questions is impractical—if not infeasible—due to cost, the level of personalization, or ethical concerns. Observational data offer a valuable alternative, but their lack of explicit randomization makes statistical analysis particularly challenging. In this thesis, we exploit the richness of modern observational data to develop methods for personalized causal inference. In the first part, we introduce a framework for causal inference using exponential family modeling. In particular, we reduce answering causal questions to learning exponential family from one sample. En route, we introduce a computationally tractable alternative to maximum likelihood estimation for learning exponential family. In the second part, we leverage ideas from doubly robust estimation to enable causal inference with black-box matrix completion under a latent factor model.
Date issued
2025-02Department
Massachusetts Institute of Technology. Department of Electrical Engineering and Computer SciencePublisher
Massachusetts Institute of Technology