Robot Fleet Learning From Heterogeneous Data
Author(s)
Wang, Lirui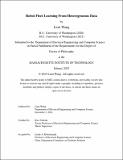
DownloadThesis PDF (56.45Mb)
Advisor
Tedrake, Russ
Terms of use
Metadata
Show full item recordAbstract
One of the key roadblocks for training generalist robotic models today is heterogeneity. Previous robot learning methods often collect data to train with one specific embodiment for one task, which is expensive and prone to overfitting. Similar to humans, robots and embodied agents inherently have to deal with heterogeneous inputs and outputs due to the nature of the perception-action loops across diverse environments. The data format and distributions collected from these systems and used for training them are varied in different modalities such as color, depth, tactile, and proprioceptive information, and/or collected in different domains such as simulation, real robots, and human videos. Moreover, fleets of robots and machines ingest massive amounts of streaming data generated by interacting with their environments in a distributed fashion, and teams of robots shall co-acquire diverse skills through their experiences in varied settings. The core idea behind my research, fleet learning, is to embrace the heterogeneous nature of robot learning to develop efficient and general algorithms. In this thesis, I will present a few angles toward tackling such challenging problems and application domains, ranging from tokenizing data, aligning representations, and merging policies, to composing skills. We develop insights and theories, often from linear settings, for how fleet learning can lead to more principled and effective use of robotic data and propose algorithmic progress, often through alignments, toward building generalist robotic foundation models. Empirically, we show advanced robotic manipulation capabilities by leveraging data from multimodal sensory inputs and multiple domains. In addition to outperforming several previous state-of-the-art across simulation and real-world benchmarks, we develop intelligent systems for robotic applications such as package handling in warehouses as well as dexterous tool-use tasks that have applications such as manufacturing, logistics, and household robots.
Date issued
2025-02Department
Massachusetts Institute of Technology. Department of Electrical Engineering and Computer SciencePublisher
Massachusetts Institute of Technology