Building World Models with Neural Physics
Author(s)
Ma, Pingchuan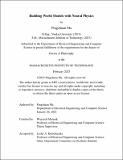
DownloadThesis PDF (13.03Mb)
Advisor
Matusik, Wojciech
Terms of use
Metadata
Show full item recordAbstract
World models learn the dynamics of environments in a data-driven manner, enhancing performance and efficiency in downstream tasks such as control, design, recognition, and generation, thanks to cost-effective simulation and differentiability. A pre-trained world model should ideally (1) accurately simulate ground-truth dynamics, (2) adapt easily to novel configurations, and (3) generalize across diverse physical effects. Previous attempts in this area have either utilized differentiable model-based physics with few parameters exposed or trained for specific scenarios with minimal physical priors integrated. These world models fall short of their objectives, limiting their applicability in real-world accuratecritic deployments and scalability to larger pre-trained world models. In this thesis, we aim to build world models with neural physics, a hybrid neural-physics framework that models the basic dynamics with differentiable physics while learning all additional modules through neural networks. By integrating neural physics, the world models adhere closely to physical principles while efficiently learning diverse effects. The modular structure of neural physics allows world models to generalize to novel configurations simply by installing different pretrained neural modules. We will demonstrate the effectiveness of this novel framework in applications such as reconstruction, robotic control, and scientific discovery.
Date issued
2025-02Department
Massachusetts Institute of Technology. Department of Electrical Engineering and Computer SciencePublisher
Massachusetts Institute of Technology