The SpaseCroissant Oven: Automatic Metadata Generation For Open-Source Space Weather Datasets
Author(s)
Chen, Edenna H.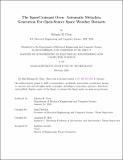
DownloadThesis PDF (1.887Mb)
Advisor
Devadas, Srini
How, Jonathan P.
Terms of use
Metadata
Show full item recordAbstract
The rise of machine learning (ML) algorithms has led to a parallel rise in ML-ready datasets. A novel metadata schema released by OpenAI and MLCommons called Croissant, which is specifically designed for ML-ready datasets, aims to increase data accessibility, user understanding of data, and accuracy of claims based on data. However, current methods to automatically generate Croissant metadata present difficulties, such as involving manual entries. This can be especially difficult when attempting to preserve information about large ML-ready datasets, which are often derived from large scientific repositories belonging to organizations such as National Aeronautics and Space Administration (NASA). These major scientific repositories provide their own metadata standards, such as NASA’s Space Physics Archive Search and Extract (SPASE) schema but context from this metadata can often be lost during data processing. This thesis presents a novel, improved approach to Croissant metadata generation which involves a hybrid parsing logic and Large Language Model (LLM) inference approach, as well as recommendations for future Croissant standards and SPASE to Croissant schema metadata conversion, that aims to retain this lost context.
Date issued
2025-02Department
Massachusetts Institute of Technology. Department of Electrical Engineering and Computer SciencePublisher
Massachusetts Institute of Technology