Real-time analysis of physiological data and development of alarm algorithms for patient monitoring in the Intensive Care Unit
Author(s)
Zhang, Ying, 1976-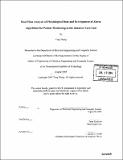
DownloadFull printable version (6.048Mb)
Other Contributors
Massachusetts Institute of Technology. Dept. of Electrical Engineering and Computer Science.
Advisor
Peter Szolovits.
Terms of use
Metadata
Show full item recordAbstract
The lack of effective data integration and knowledge representation in patient monitoring limits its utility to clinicians. Intelligent alarm algorithms that use artificial intelligence techniques have the potential to reduce false alarm rates and to improve data integration and knowledge representation. Crucial to the development of such algorithms is a well-annotated data set. In previous studies, clinical events were either unavailable or annotated without accurate time synchronization with physiological signals, generating uncertainties during both the development and evaluation of intelligent alarm algorithms. This research aims to help eliminate these uncertainties by designing a system that simultaneously collects physiological data and clinical annotations at the bedside, and to develop alarm algorithms in real time based on patient-specific data collected while using this system. In a standard pediatric intensive care unit, a working prototype of this system has helped collect a dataset of 196 hours of vital sign measurements at 1 Hz with 325 alarms generated by the bedside monitor and 2 instances of false negatives. About 89% of these alarms were clinically relevant true positives; 6% were true positives without clinical relevance; and 5% were false positives. Real-time machine learning showed improved performance over time and generated alarm algorithms that outperformed the previous generation of bedside monitors and came close in performance to the new generation. Results from this research suggest that the alarm algorithm(s) of the new patient monitoring systems have significantly improved sensitivity and specificity. They also demonstrated the feasibility of real-time learning at the bedside. Overall, they indicate (cont.) that the methods developed in this research have the potential of helping provide patient-specific decision support for critical care.
Description
Thesis (M. Eng.)--Massachusetts Institute of Technology, Dept. of Electrical Engineering and Computer Science, 2003. Includes bibliographical references (p. 86-90).
Date issued
2003Department
Massachusetts Institute of Technology. Department of Electrical Engineering and Computer SciencePublisher
Massachusetts Institute of Technology
Keywords
Electrical Engineering and Computer Science.