Smoothed analysis of Gaussian elimination
Author(s)
Sankar, Arvind, 1976-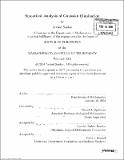
DownloadFull printable version (2.497Mb)
Other Contributors
Massachusetts Institute of Technology. Dept. of Mathematics.
Advisor
Daniel A. Spielman.
Terms of use
Metadata
Show full item recordAbstract
We present a smoothed analysis of Gaussian elimination, both with partial pivoting and without pivoting. Let A be any matrix and let A be a slight random perturbation of A. We prove that it is unlikely that A has large condition number. Using this result, we prove it is unlikely that A has large growth factor under Gaussian elimination without pivoting. By combining these results, we bound the smoothed precision needed to perform Gaussian elimination without pivoting. Our results improve the average-case analysis of Gaussian elimination without pivoting performed by Yeung and Chan (SIAM J. Matrix Anal. Appl., 1997). We then extend the result on the growth factor to the case of partial pivoting, and present the first analysis of partial pivoting that gives a sub-exponential bound on the growth factor. In particular, we show that if the random perturbation is Gaussian with variance [sigma]², then the growth factor is bounded by (n/[sigma])[to the power of] (o log n) with very high probability.
Description
Thesis (Ph. D.)--Massachusetts Institute of Technology, Dept. of Mathematics, 2004. Includes bibliographical references (p. 59-60).
Date issued
2004Department
Massachusetts Institute of Technology. Department of MathematicsPublisher
Massachusetts Institute of Technology
Keywords
Mathematics.