Probabilistic state estimation in regimes of nonlinear error growth
Author(s)
Lawson, W. Gregory, 1975-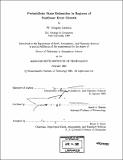
DownloadFull printable version (40.39Mb)
Other Contributors
Massachusetts Institute of Technology. Technology, Dept. of Earth, Atmospheric, and Planetary Sciences.
Advisor
James A. Hansen.
Terms of use
Metadata
Show full item recordAbstract
State estimation, or data assimilation as it is often called, is a key component of numerical weather prediction (NWP). Nearly all implementable methods of state estimation suitable for NWP are forced to assume that errors remain in regimes of linear error growth and retain distributions of Gaussian uncertainty, yet nonlinear systems like the atmosphere can readily allow regimes of nonlinear error growth and, in turn, produce distributions of non- Gaussian uncertainty. State-of-the-art, ensemble-based methods of state estimation suitable for NWP are examined to gauge the consequences and relevance of violating the linear error growth assumption. For quite generic sources of non-Gaussian uncertainty, the methods are observed to fail, as they must, and the obtained analyses become probabilistically unreliable before becoming inaccurate. The mispositioning of coherent features is identified as a specific, geophysically relevant source of non-Gaussian uncertainty that can easily cause the state-of-the-art methods of state estimation to fail. However, an understanding of relevant phenomenology sometimes allows these same methods to remain successful owing to an available redefinition of the involved errors. The redefinition is phrased as an alternative error model. It is recognized and exploited that non-Gaussian additive Eulerian errors can come from Gaussian Lagrangian position errors. A two-step, augmented state vector approach is developed that is suitable for use with coherent features and that relies only on implementable methods of state estimation. (cont.) By combining the dual Eulerian and Lagrangian state information into one vector, an ensemble can approximate their covariance, thus allowing each component's uncertainty to be reduced. The first step of the two-step approach reduces the feature position errors in an effort to render the residual additive errors Gaussian, thereby allowing the second step of an implementable state estimation method to proceed successfully. Philosophically, the two-step approach uses physical knowledge of the problem (as phrased by the error model) to compensate for neglected important non-Gaussian uncertainty structure in the state estimation process. The proposed two-step approach successfully allows use of implementable methods of state estimation to obtain probabilistically reliable analyses in regimes of nonlinear error growth, something unavailable using current standards.
Description
Thesis (Ph. D.)--Massachusetts Institute of Technology, Dept. of Earth, Atmospheric, and Planetary Sciences, 2005. Includes bibliographical references (p. 273-286).
Date issued
2005Department
Massachusetts Institute of Technology. Department of Earth, Atmospheric, and Planetary SciencesPublisher
Massachusetts Institute of Technology
Keywords
Technology, Earth, Atmospheric, and Planetary Sciences.