Extended discrete choice models : integrated framework, flexible error structures, and latent variables
Author(s)
Walker, Joan Leslie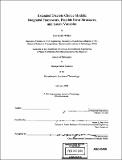
DownloadFull printable version (12.48Mb)
Other Contributors
Massachusetts Institute of Technology. Dept. of Civil and Environmental Engineering.
Advisor
Moshe E. Ben-Akiva.
Terms of use
Metadata
Show full item recordAbstract
Discrete choice methods model a decision-maker's choice among a set of mutually exclusive and collectively exhaustive alternatives. They are used in a variety of disciplines (transportation, economics, psychology, public policy, etc.) in order to inform policy and marketing decisions and to better understand and test hypotheses of behavior. This dissertation is concerned with the enhancement of discrete choice methods. The workhorses of discrete choice are the multinomial and nested logit models. These models rely on simplistic assumptions, and there has been much debate regarding their validity. Behavioral researchers have emphasized the importance of amorphous influences on behavior such as context, knowledge, and attitudes. Cognitive scientists have uncovered anomalies that appear to violate the microeconomic underpinnings that are the basis of discrete choice analysis. To address these criticisms, researchers have for some time been working on enhancing discrete choice models. While there have been numerous advances, typically these extensions are examined and applied in isolation. In this dissertation, we present, empirically demonstrate, and test a generalized methodological framework that integrates the extensions of discrete choice. The basic technique for integrating the methods is to start with the multinomial logit formulation, and then add extensions that relax simplifying assumptions and enrich the capabilities of the basic model. The extensions include: - Specifying factor analytic (probit-like) disturbances i order to provide a flexible covariance structure, thereby relaxing the IIA condition and enabling estimation of unobserved heterogeneity through techniques such as random parameters. - Combining revealed and stated preferences in order to draw on the advantages of both types of data, thereby reducing bias and improving efficiency of the parameter estimates. - Incorporating latent variables in order to provide a richer explanation of behavior by explicitly representing the formation and effects of latent constructs such as attitudes and perceptions. - Stipulating latent classes in order to capture latent segmentation, for example. in terms of taste parameters, choice sets, and decision protocols. The guiding philosophy is that the generalized framework allows for a more realistic representation of the behavior inherent in the choice process, and consequently a better understanding of behavior, improvements in forecasts, and valuable information regarding the validity of simpler model structures. These generalized models often result in functional forms composed of complex multidimensional integrals. Therefore a key aspect of the framework is its 'logit kernel' formulation in which the disturbance of the choice model includes an additive i.i.d Gumbel term. This formulation can replicate all known error structures (as we show here) and it leads to a straightforward probability simulator (of a multinomial logit form) for use in maximum simulated likelihood estimation. The proposed framework and suggested implementation leads to a flexible, tractable, theoretically grounded, empirically verifiable. and intuitive method for incorporating and integrating complex behavioral processes in the choice model. In addition to the generalized framework, contributions are also made to two of the key methodologies hat make up the framework. First, we present new results regarding identification and normalization of he disturbance parameters of a logit kernel model. n particular, we show that identification is not always intuitive, it is not always analogous to the systematic portion. and it is not necessarily like probit. Second. we present a general framework and methodology for incorporating latent variables into choice models via the integration of choice and latent variable models and the use of psychometric data (for example. responses to attitudinal survey questions). Throughout the dissertation, empirical results are presented to highlight findings and to empirically demonstrate and test the generalized framework. The impact of the extensions cannot be known a priori. and the only way to test their value (as well as the validity of a simpler model structure) is to estimate the complex models. Sometimes the extensions result in large improvements in fit as well as in more satisfying behavioral representations. Conversely, sometimes the extensions have marginal impact. thereby showing that the more parsimonious structures are robust. All methods are often not necessary. and the generalized framework provides an approach for developing the best model specification that makes use of available data and is reflective of behavioral hypotheses.
Description
Thesis (Ph.D.)--Massachusetts Institute of Technology, Dept. of Civil and Environmental Engineering, 2001. Includes bibliographical references (leaves 199-209).
Date issued
2001Department
Massachusetts Institute of Technology. Department of Civil and Environmental EngineeringPublisher
Massachusetts Institute of Technology
Keywords
Civil and Environmental Engineering.