Applications of the Bayesian approach for experimentation and estimation
Author(s)
De Man, Patrick A. P. (Patrick Antonius Petrus)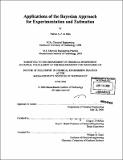
DownloadFull printable version (27.35Mb)
Other Contributors
Massachusetts Institute of Technology. Dept. of Chemical Engineering.
Advisor
Gregory J. McRae.
Terms of use
Metadata
Show full item recordAbstract
A Bayesian framework for systematic data collection and parameter estimation is proposed to aid experimentalists in effectively generating and interpreting data. The four stages of the Bayesian framework are: system description, system analysis, experimentation, and estimation. System description consists of specifying the system under investigation and collecting available information for the parameter estimation. Subsequently, system analysis entails a more in-depth system study by implementing various mathematical tools such as an observability and sensitivity analysis. The third stage in the framework is experimentation, consisting of experimental design, system calibration, and performing actual experiments. Finally, the last stage is estimation, where all relevant information and collected data is used for estimating the desired quantities. The Bayesian approach embedded within this framework provides a versatile, robust, and unified methodology allowing for consistent incorporation and propagation of uncertainty. To demonstrate the benefits, the Bayesian framework was applied to two different case studies of complex reaction engineering problems. (cont.) The first case study involved the estimation of a kinetic rate parameter in a system of coupled chemical reactions involving the relaxation of the reactive O(D) oxygen atom. The second case study was aimed at estimating multiple kinetic rate parameters concurrently to gain an understanding regarding the reaction mechanism of the oxygen addition to the transient cyclohexadienyl radical. An important advantage of the proposed Bayesian framework demonstrated with these case studies is the possibility of 'real-time' updating of the state of knowledge regarding the parameter estimate allowing for exploitation of the close relationship between experimentation and estimation. This led to identifying systematic errors among experiments and devising a stopping rule for experimentation based on incremental information gain per experiment. Additional advantages were the improved understanding of the underlying reaction mechanism, identification of experimental outliers, and more precisely estimated parameters. (cont.) A unique feature of this work is the use of Markov Chain Monte Carlo simulations to overcome the computational problems affecting previous applications of the Bayesian approach to complex engineering problems. Traditional restricting assumptions can therefore be relaxed so that the case studies could involve non-Gaussian distributions, applied to multi-dimensional, nonlinear systems.
Description
Thesis (Ph. D.)--Massachusetts Institute of Technology, Dept. of Chemical Engineering, 2006. Page 272 blank. Includes bibliographical references (p. 267-271).
Date issued
2006Department
Massachusetts Institute of Technology. Department of Chemical EngineeringPublisher
Massachusetts Institute of Technology
Keywords
Chemical Engineering.