Recovering sample diversity in Rao-Blackwellized particle filters for simultaneous localization and mapping
Author(s)
Anderson, Andrew D. (Andrew David)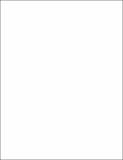
DownloadFull printable version (16.41Mb)
Alternative title
Recovering sample diversity in RDPF for SLAMI
Other Contributors
Massachusetts Institute of Technology. Dept. of Aeronautics and Astronautics.
Advisor
Don Gustafson and John Deyst.
Terms of use
Metadata
Show full item recordAbstract
This thesis considers possible solutions to sample impoverishment, a well-known failure mode of the Rao-Blackwellized particle filter (RBPF) in simultaneous localization and mapping (SLAMI) situations that arises when precise feature measurements yield a limited perceptual distribution relative to a motion-based proposal distribution. One set of solutions propagates particles according to a more advanced proposal distribution that includes measurement information. Other methods recover lost sample diversity by resampling particles according to a continuous distribution formed by regularization kernels. Several advanced proposals and kernel shaping regularization methods are considered based on the RBPF and tested in a Monte Carlo simulation involving an agent traveling in an environment and observing uncertain landmarks. RMS error of range-bearing feature measurements was reduced to evaluate performance during proposal-perceptual distribution mismatch. A severe loss in accuracy due to sample impoverishment is seen in the standard RBPF at a measurement range RMS error of 0.001 m in a 10 m x 10 m environment. (cont.) Results reveal a robust and accurate solution to sample impoverishment in an RBPF with an added fixed-variance regularization algorithm. This algorithm produced an average 0.05 m improvement in agent pose CEP over standard FastSLAM 1.0 and a 0.1 m improvement over an RBPF that includes feature observations in formulation of a proposal distribution. This algorithm is then evaluated in an actual SLAM environment with data from a Swiss Ranger LIDAR measurement device and compared alongside an extended Kalman filter (EKF) based SLAM algorithm. Pose error is immediately recovered in cases of a 1.4 m error in initial agent uncertainty using the improved FastSLAM algorithm, and it continues to maintain an average 0.75 m improvement over an EKF in pose CEP through the scenario.
Description
Thesis (S.M.)--Massachusetts Institute of Technology, Dept. of Aeronautics and Astronautics, 2006. Includes bibliographical references (p. 105-109).
Date issued
2006Department
Massachusetts Institute of Technology. Department of Aeronautics and AstronauticsPublisher
Massachusetts Institute of Technology
Keywords
Aeronautics and Astronautics.