Inferring noncompensatory choice heuristics
Author(s)
Yee, Michael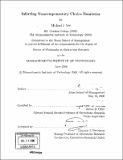
DownloadFull printable version (5.753Mb)
Other Contributors
Massachusetts Institute of Technology. Operations Research Center.
Advisor
James B. Orlin.
Terms of use
Metadata
Show full item recordAbstract
Human decision making is a topic of great interest to marketers, psychologists, economists, and others. People are often modeled as rational utility maximizers with unlimited mental resources. However, due to the structure of the environment as well as cognitive limitations, people frequently use simplifying heuristics for making quick yet accurate decisions. In this research, we apply discrete optimization to infer from observed data if a person is behaving in way consistent with a choice heuristic (e.g., a noncompensatory lexicographic decision rule). We analyze the computational complexity of several inference related problems, showing that while some are easy due to possessing a greedoid language structure, many are hard and likely do not have polynomial time solutions. For the hard problems we develop an exact dynamic programming algorithm that is robust and scalable in practice, as well as analyze several local search heuristics. We conduct an empirical study of SmartPhone preferences and find that the behavior of many respondents can be explained by lexicographic strategies. (cont.) Furthermore, we find that lexicographic decision rules predict better on holdout data than some standard compensatory models. Finally, we look at a more general form of noncompensatory decision process in the context of consideration set formation. Specifically, we analyze the computational complexity of rule-based consideration set formation, develop solution techniques for inferring rules given observed consideration data, and apply the techniques to a real dataset.
Description
Thesis (Ph. D.)--Massachusetts Institute of Technology, Sloan School of Management, Operations Research Center, 2006. Includes bibliographical references (p. 121-128).
Date issued
2006Department
Massachusetts Institute of Technology. Operations Research Center; Sloan School of ManagementPublisher
Massachusetts Institute of Technology
Keywords
Operations Research Center.