Evolutionary, developmental neural networks for robust robotic control
Author(s)
Adams, Bryan (Bryan Paul), 1977-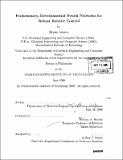
DownloadFull printable version (13.19Mb)
Other Contributors
Massachusetts Institute of Technology. Dept. of Electrical Engineering and Computer Science.
Advisor
Rodney A. Brooks.
Terms of use
Metadata
Show full item recordAbstract
The use of artificial evolution to synthesize controllers for physical robots is still in its infancy. Most applications are on very simple robots in artificial environments, and even these examples struggle to span the "reality gap," a name given to the difference between the performance of a simulated robot and the performance of a.real robot using the same evolved controller. This dissertation describes three methods for improving the use of artificial evolution as a tool for generating controllers for physical robots. First, the evolutionary process must incorporate testing on the physical robot. Second, repeated structure on the robot should be exploited. Finally, prior knowledge about the robot and task should be meaningfully incorporated. The impact of these three methods, both in simulation and on physical robots, is demonstrated, quantified, and compared to hand-designed controllers.
Description
Thesis (Ph. D.)--Massachusetts Institute of Technology, Dept. of Electrical Engineering and Computer Science, 2006. Includes bibliographical references (p. 136-143).
Date issued
2006Department
Massachusetts Institute of Technology. Department of Electrical Engineering and Computer SciencePublisher
Massachusetts Institute of Technology
Keywords
Electrical Engineering and Computer Science.