Optimization under uncertainty in radiation therapy
Author(s)
Chan, Timothy Ching-Yee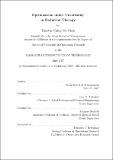
DownloadFull printable version (3.625Mb)
Other Contributors
Massachusetts Institute of Technology. Operations Research Center.
Advisor
John N. Tsitsiklis.
Terms of use
Metadata
Show full item recordAbstract
In the context of patient care for life-threatening illnesses, the presence of uncertainty may compromise the quality of a treatment. In this thesis, we investigate robust approaches to managing uncertainty in radiation therapy treatments for cancer. In the first part of the thesis, we study the effect of breathing motion uncertainty on intensity-modulated radiation therapy treatments of a lung tumor. We construct a robust framework that generalizes current mathematical programming formulations that account for motion. This framework gives insight into the trade-off between sparing the healthy tissues and ensuring that the tumor receives sufficient dose. With this trade-off in mind, we show that our robust solution outperforms a nominal (no uncertainty) solution and a margin (worst-case) solution on a clinical case. Next, we perform an in-depth study into the structure of different intensity maps that were witnessed in the first part of the thesis. We consider parameterized intensity maps and investigate their ability to deliver a sufficient dose to the tumor in the presence of motion that follows a Gaussian distribution. We characterize the structure of optimal intensity maps in terms of certain conditions on the problem parameters. (cont.) Finally, in the last part of the thesis, we study intensity-modulated proton therapy under uncertainty in the location of maximum dose deposited by the beamlets of radiation. We provide a robust formulation for the optimization of proton-based treatments and show that it outperforms traditional formulations in the face of uncertainty. In our computational experiments, we see evidence that optimal robust solutions use the physical characteristics of the proton beam to create dose distributions that are far less sensitive to the underlying uncertainty.
Description
Thesis (Ph. D.)--Massachusetts Institute of Technology, Sloan School of Management, Operations Research Center, 2007. This electronic version was submitted by the student author. The certified thesis is available in the Institute Archives and Special Collections. Includes bibliographical references (p. 175-182).
Date issued
2007Department
Massachusetts Institute of Technology. Operations Research Center; Sloan School of ManagementPublisher
Massachusetts Institute of Technology
Keywords
Operations Research Center.