Large-scale dynamic observation planning for unmanned surface vessels
Author(s)
Miller, John V. (John Vaala)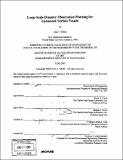
DownloadFull printable version (31.34Mb)
Other Contributors
Massachusetts Institute of Technology. Operations Research Center.
Advisor
James B. Orlin.
Terms of use
Metadata
Show full item recordAbstract
With recent advances in research and technology, autonomous surface vessel capabilities have steadily increased. These autonomous surface vessel technologies enable missions and tasks to be performed without the direction of human operators, and have changed the way scientists and engineers approach problems. Because these robotic devices can work without manned guidance, they can execute missions that are too difficult, dangerous, expensive, or tedious for human operators to attempt. The United States government is currently expanding the use of autonomous surface vessel technologies through the United States Navy's Spartan Scout unmanned surface vessel (USV) and NASA's Ocean-Atmosphere Sensor Integration System (OASIS) USV. These USVs are well-suited to complete monotonous, dangerous, and time-consuming missions. The USVs provide better performance, lower cost, and reduced risk to human life than manned systems. In this thesis, we explore how to plan multiple USV observation schedules for two significant notional observation scenarios, collecting water temperatures ahead of the path of a hurricane, and collecting fluorometer readings to observe and track a harmful algal bloom. (cont.) A control system must be in place that coordinates a fleet of USVs to targets in an efficient manner. We develop three algorithms to solve the unmanned surface vehicle observation-planning problem. A greedy construction heuristic runs fastest, but produces suboptimal plans; a 3-phase algorithm which combines a greedy construction heuristic with an improvement phase and an insertion phase, requires more execution time, but generates significantly better plans; an optimal mixed integer programming algorithm produces optimal plans, but can only solve small problem instances.
Description
Thesis (S.M.)--Massachusetts Institute of Technology, Sloan School of Management, Operations Research Center, 2007. Includes bibliographical references (p. 129-134).
Date issued
2007Department
Massachusetts Institute of Technology. Operations Research Center; Sloan School of ManagementPublisher
Massachusetts Institute of Technology
Keywords
Operations Research Center.