Data-driven revenue management
Author(s)
Uichanco, Joline Ann Villaranda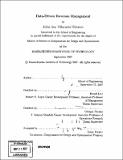
DownloadFull printable version (4.692Mb)
Other Contributors
Massachusetts Institute of Technology. Computation for Design and Optimization Program.
Advisor
Retsef Levi and Georgia Perakis.
Terms of use
Metadata
Show full item recordAbstract
In this thesis, we consider the classical newsvendor model and various important extensions. We do not assume that the demand distribution is known, rather the only information available is a set of independent samples drawn from the demand distribution. In particular, the variants of the model we consider are: the classical profit-maximization newsvendor model, the risk-averse newsvendor model and the price-setting newsvendor model. If the explicit demand distribution is known, then the exact solutions to these models can be found either analytically or numerically via simulation methods. However, in most real-life settings, the demand distribution is not available, and usually there is only historical demand data from past periods. Thus, data-driven approaches are appealing in solving these problems. In this thesis, we evaluate the theoretical and empirical performance of nonparametric and parametric approaches for solving the variants of the newsvendor model assuming partial information on the distribution. For the classical profit-maximization newsvendor model and the risk-averse newsvendor model we describe general non-parametric approaches that do not make any prior assumption on the true demand distribution. We extend and significantly improve previous theoretical bounds on the number of samples required to guarantee with high probability that the data-driven approach provides a near-optimal solution. By near-optimal we mean that the approximate solution performs arbitrarily close to the optimal solution that is computed with respect to the true demand distributions. (cont.) For the price-setting newsvendor problem, we analyze a previously proposed simulation-based approach for a linear-additive demand model, and again derive bounds on the number of samples required to ensure that the simulation-based approach provides a near-optimal solution. We also perform computational experiments to analyze the empirical performance of these data-driven approaches.
Description
Thesis (S.M.)--Massachusetts Institute of Technology, Computation for Design and Optimization Program, 2007. Includes bibliographical references (p. 125-127).
Date issued
2007Department
Massachusetts Institute of Technology. Computation for Design and Optimization ProgramPublisher
Massachusetts Institute of Technology
Keywords
Computation for Design and Optimization Program.