Computational studies of hydrogen storage materials and the development of related methods
Author(s)
Mueller, Timothy Keith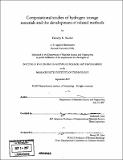
DownloadFull printable version (53.66Mb)
Other Contributors
Massachusetts Institute of Technology. Dept. of Materials Science and Engineering.
Advisor
Gerbrand Ceder.
Terms of use
Metadata
Show full item recordAbstract
Computational methods, including density functional theory and the cluster expansion formalism, are used to study materials for hydrogen storage. The storage of molecular hydrogen in the metal-organic framework with formula unit Zn40(02C-C6H6-COD3 is considered. It is predicted that hydrogen adsorbs at five sites near the metal-oxide cluster, in good agreement with recent experimental data. It is also shown that the metal-oxide cluster affects the electronic structure of the organic linker, qualitatively affecting the way in which hydrogen binds to the linker. Lithium imide (Li2NH), a material present in several systems being considered for atomic hydrogen storage, is extensively investigated. A variation of the cluster expansion formalism that accounts for continuous bond orientations is developed to search for the ground state structure of this material, and a structure with a calculated energy lower than any known is found. Two additional discrete cluster expansions are used to predict that the experimentally observed phase of lithium imide is metastable at temperatures below approximately 200 K and stabilized primarily by vibrational entropy at higher temperatures. A new structure for this low-temperature phase that agrees well with experimental data is proposed. A method to improve the predictive power of cluster expansions through the application of statistical learning theory is developed, as are related algorithms. The Bayesian approach to regularization is used to show that by taking advantage of the prior expectation that cluster expansions are local, the convergence and prediction properties of cluster expansions can be significantly improved. (cont.) A variety of methods to generate cluster expansions are evaluated on three different binary systems. It is suggested that a good method to generate cluster expansions is to use a prior distribution that penalizes the ECI for larger clusters more and has few parameters. It is shown that the generalized cross-validation score can be an efficient and effective substitute for the leave-one-out cross-validation score when searching for a good set of parameters for the prior distribution. Finally it is shown that the Bayesian approach can also be used to improve the convergence and prediction properties of cluster expansions for surfaces, nanowires, nanoparticles, and certain defects.
Description
Thesis (Ph. D.)--Massachusetts Institute of Technology, Dept. of Materials Science and Engineering, 2007. Includes bibliographical references (p. 193-199).
Date issued
2007Department
Massachusetts Institute of Technology. Department of Materials Science and EngineeringPublisher
Massachusetts Institute of Technology
Keywords
Materials Science and Engineering.