Large scale disease prediction
Author(s)
Schmid, Patrick R. (Patrick Raphael)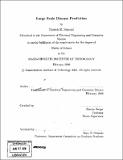
DownloadFull printable version (6.677Mb)
Other Contributors
Massachusetts Institute of Technology. Dept. of Electrical Engineering and Computer Science.
Advisor
Bonnie Berger.
Terms of use
Metadata
Show full item recordAbstract
The objective of this thesis is to present the foundation of an automated large-scale disease prediction system. Unlike previous work that has typically focused on a small self-contained dataset, we explore the possibility of combining a large amount of heterogeneous data to perform gene selection and phenotype classification. First, a subset of publicly available microarray datasets was downloaded from the NCBI Gene Expression Omnibus (GEO) [18, 5]. This data was then automatically tagged with Unified Medical Language System (UMLS) concepts [7]. Using the UMLS tags, datasets related to several phenotypes were obtained and gene selection was performed on the expression values of this tagged microarray data. Using the tagged datasets and the list of genes selected in the previous step, classifiers that can predict whether or not a new sample is also associated with a given UMLS concept based solely on the expression data were created. The results from this work show that it is possible to combine a large heterogeneous set of microarray datasets for both gene selection and phenotype classification, and thus lays the foundation for the possibility of automatic classification of disease types based on gene expression data in a clinical setting.
Description
Thesis (S.M.)--Massachusetts Institute of Technology, Dept. of Electrical Engineering and Computer Science, 2008. Includes bibliographical references (leaves 69-73).
Date issued
2008Department
Massachusetts Institute of Technology. Department of Electrical Engineering and Computer SciencePublisher
Massachusetts Institute of Technology
Keywords
Electrical Engineering and Computer Science.